SnapLogic is an agnostic cloud integration platform as a service (iPaaS) that ties together and orchestrates data flows between on-premises apps and data, cloud SaaS applications, and a variety of cloud data warehouses. As such, we’re often asked for our opinion on which cloud data warehouse “is the best?”
Amazon Redshift vs. Google BigQuery vs. Snowflake Pricing
The answer is, as you would expect, it depends. There are many factors. For example, I previously blogged about the important factors to consider when determining whether recently-IPO’d Snowflake is a good fit for your environment.
With SnapLogic integration outside of your warehouse platform, you do not have to be locked into any one cloud data warehouse choice. Our graphical, low-code user interface enables you to easily change or mix-and-match cloud data warehouses to customize for the different needs within your organization. As a result, when comparing cloud data warehouses, an area that many of our customers specifically want to focus on is pricing.
Thus, with the objective of providing a price comparison reference tool and a blueprint to orient to your specific needs, this blog is first in a series that compares the pricing approaches for three popular cloud data warehouse options (see Table 1): Amazon Redshift, Google BigQuery, and Snowflake.
While all three are excellent choices, with unique advantages, each cloud data warehouse vendor approaches pricing very differently. It can be confusing.
If you’re already familiar with cloud data warehousing pricing structures, but need a reference tool to share with others or to include in RFPs, this information is useful to know:
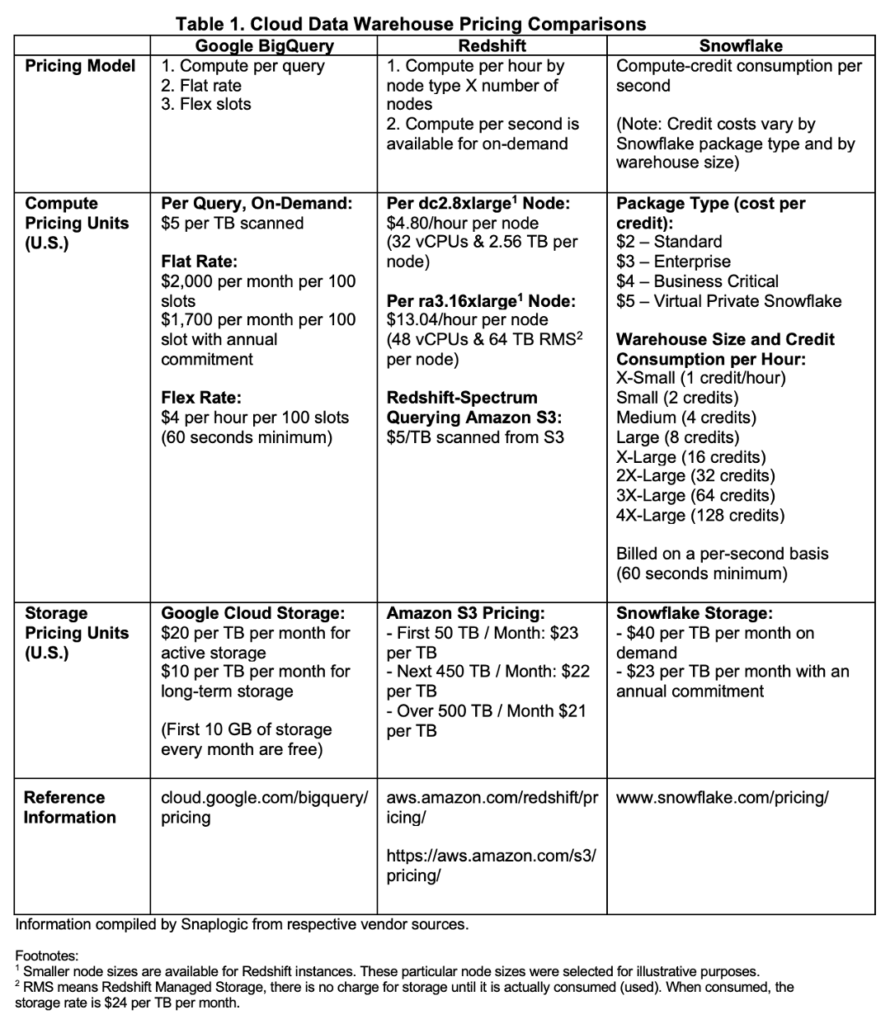
As you can see, pricing approaches vary greatly. Even if you run your own benchmark tests against your own data and query requirements, you’ll still want to know how pricing differs between each platform as queries and data change or grow.
Challenges When Comparing Pricing Based On vCPUs and Nodes
Many IT shops attempt to conduct comparisons based on a specific configuration, such as the number of vCPUs and memory, etc. Yet, from a price perspective, this is not apples-to-apples because vCPU means something different for each cloud data warehouse. Plus, cloud data warehouse vendors produce a performance in different ways (e.g., by utilizing query data pruning, compute separation, etc.), thereby making the vCPU countless meaningful as a measure to equate pricing.
For example, according to Google Big Query documentation, a “slot” is defined as a virtual CPU. Without any other supporting information, it’s difficult to know the exact detail of a BigQuery slot other than it represents a unit of computing within its massive server infrastructure. Based on our experience, we advise to not equate or expect a “500 vCPU” (i.e., 500 slots) BigQuery service to perform as well as, for example, a 500 vCPU Redshift environment (dc2.8xlarge, ra3.16xlarge,…). The price difference is also dramatic.
Lastly, vCPU information may not be publicly available, as is the case with Snowflake, which chooses to cloak this detail behind its cloud data warehouse service.
Comparing prices based on node count is similar to comparing vCPUs – care must be taken to determine how node count is meaningful. Google BigQuery does not employ the concept of nodes and Snowflake does not directly publicly disclose node-count specifics. Although, note that Snowflake documentation indicates the “X-Small” size is a single “server”, and the server count doubles up to a maximum of 128 servers, which is a Snowflake 4X-Large.
This said a Snowflake server should not be equated directly to a Redshift node because the underlying node types may be different and compute methodologies vary.
Illustrating Pricing Differences
As mentioned, it can be confusing to directly compare prices. The ideal, of course, is to run your own tests across the different cloud data warehouses to get a feel for pricing. Still, to avoid unexpected billing surprises, it is beneficial to be aware of how each data warehouse operates and generates compute and storage charges. Especially now given the growing popularity of pause and resume features, per-second billing, and related dependencies.
With the next blog in this series, we will look at hypothetical scenarios that provide a guide to pricing.