Data transformation reconciles and standardizes data so that it’s useful as a single source of “data truth.” And for decades, the transformation and migration method of choice has been ETL (extract, transform, load). Organizations using on-premise data storage often paid a premium for extra space and processing power, so it made sense for them to clean and transform their data before loading it to storage.
Today, thanks to the rise of cloud storage, we have a second option: ELT (extract, load, transform). Organizations that have switched to the cloud are no longer constrained by size and processing power, so many now choose to transform their data after loading it to storage.
Does that mean ELT is the more favorable process? Often, but not always. Read on to learn the functional differences between the two processes and explore their respective considerations and use cases.
What is ETL?
ETL stands for “Extract, Transform, and Load” and is the process of extracting data from one or more sources and moving it to a staging environment. There, the data is cleaned and transformed before being loaded into a data warehouse for storage and analysis.
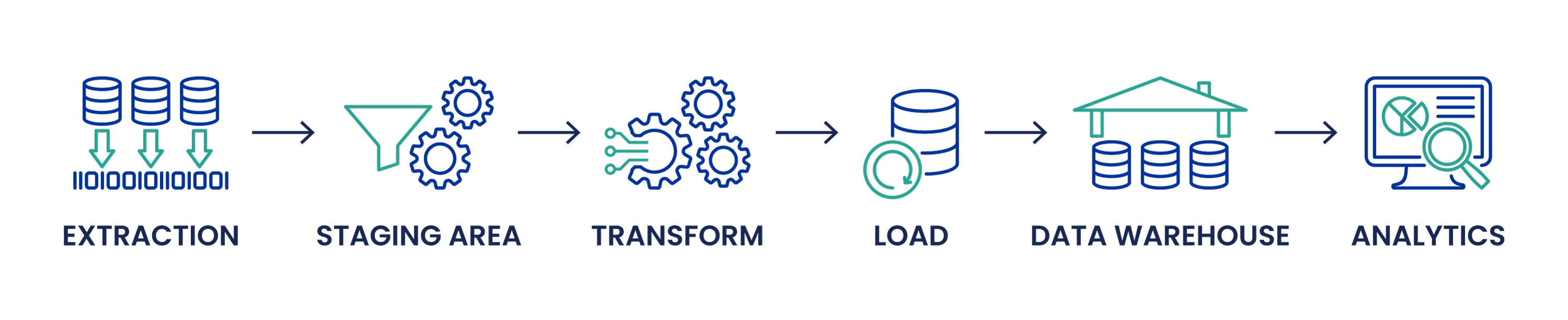
Here’s a breakdown of the three ETL stages:
Extract
- Data is extracted from source(s) and moved to a staging area. Common data-source formats include relational databases, XML, JSON, and flat files, but can include non-relational databases like Informational Management Systems too.
- The data is validated upon extraction to ensure its accuracy. Data that fails validation rules will be rejected, then discarded or (ideally) returned to its source for further diagnostics.
Transform
- The validated data is cleansed in the staging area. This crucial part of the data transformation process involves identifying corrupt, duplicate, irrelevant, noisy, or misrepresentative data and then replacing, modifying, or deleting it.
- Other transformations occur so the data can be stored in a useful form. Common transformations include sorting and filtering, merging data from multiple sources, combining or splitting rows and columns, translating coded values, and performing basic calculations. Sensitive data is also scrubbed, encrypted, redacted, and protected before it is exposed to business users.
Load
- The data is loaded to its end target for storage. For ETL, the end target is usually a data warehouse, but it can be any data store. The process for loading the data varies wildly according to organizational needs. Those that don’t rely on historical data may overwrite old data with the new information, while others may wish to create a history by loading the data in historical form at regular intervals.
- Constraints defined within the database may also trigger upon load, further filtering the data. The database may filter out duplicates that already exist in the database, reject data that’s missing mandatory fields, or perform other actions based on the parameters set by the organization.
- The stored data is now ready for further analysis. Popular data analytics tools include Tableau, Microsoft Power BI, and Qlik Sense.
The driving goal behind ETL is to load clean, consistent data to the warehouse. Loading only the necessary data frees up storage space and processing power, so it’s a good choice for organizations that store their data on-premise or use another storage solution that doesn’t scale well. Most traditional ETL tools are geared toward on-premise databases.
What is ELT?
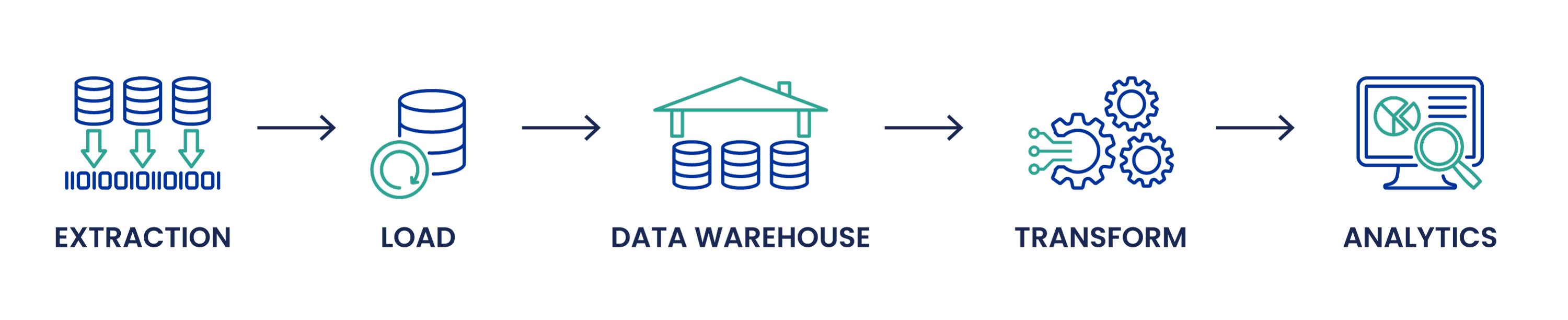
In the ELT process, the data is loaded into storage before any transformations take place.
ELT uses cloud data warehouses to load all types of data without having to make complex transformations first. This process is possible through data lakes, a special type of storage where any raw information can be uploaded. After the data has reached its target destination, it can be selected and transformed as needed.
In ELT, the business captures all data — whether they’ll use it or not — so nobody has to spend time transforming data that won’t ultimately be useful.
ELT’s speed and scalability are made possible by modern cloud server technology. By utilizing cloud-based data warehouses, businesses gain exponential storage capabilities and can drastically scale their processing power. Platforms like Amazon Redshift and Snowflake have immense processing abilities and make ELT pipelines possible.
ELT does not work with on-premise systems.
Extract
- Data is extracted from source(s) and moved to a staging area. Unlike ETL, the data doesn’t undergo a validation process at this stage.
Load
- The data is immediately loaded in its raw format to the data lake, where it will be warehoused. Popular cloud storage solutions include Amazon Web Services, Cloudera, Google Cloud, and Microsoft Azure.
Transform
- The data is transformed on an as-needed basis. This saves time in the long run because people won’t be applying transformations to data they don’t need.
Pros and cons of ETL vs. ELT
When you’re deciding whether ETL or ELT is right for your organization, the main factors to consider are the cost and complexity of your data storage. ELT is faster, as it relies on cloud storage with data lakes that can handle a massive amount of raw, unstructured, and semi-structured data. If you’re using on-premise storage, ETL helps you avoid the cost of storing unnecessary data.
Another consideration is the sensitivity of your raw data. In ETL, sensitive data can be masked or removed during the transformation process. In ELT, all data gets sent to the warehouse — potentially exposing organizations to HIPAA, CCPA, or GDPR violations. However, it’s possible to protect sensitive data during the ELT process with encryption and proper data governance.
Ultimately, the choice comes down to the following questions: Do you need to be selective about the data you store (ETL)? Or are you willing to capture and store all your data, even if you never use it (ELT)?
Benefits of ETL
- Greater flexibility – ETL allows for more complex transformations and broader toolsets while minimizing data silos and lock-ins for your transformed data.
- Easier compliance – Sensitive data sets can be redacted or encrypted, making it easier to satisfy GDPR, HIPAA, CCPA, and other compliance standards. When you transform data before it gets to the data warehouse, you greatly reduce the risk of exposing non-compliant data.
- Wider availability – ETL has been around for 20 years; there are numerous ETL tools in the Data Integration space and ETL knowledge is a common skill set among developers.
Challenges of ETL
- Slower process – ETL requires the extra step of loading the data into a staging area before processing it.
- Greater potential for human error – Since you aren’t ingesting data and loading it directly to the warehouse, there’s more potential to load erroneous data to the warehouse. Since no transformations occur after that point, incorrect data risks being used for analysis.
Benefits of ELT
- Faster availability – ELT provides faster data availability and data loading for quicker analytics.
- Stronger focus – ELT is ideal for smaller datasets with simple transformations that have minimal impact on cloud data warehouse processing utilization.
- Lower costs – ELT is cloud-based and does not require extensive, costly hardware.
- Lower maintenance – ELT generally incorporates automated solutions, so very little maintenance is needed.
- More scalable – Since ELT is flexible and unconstrained by size, the solution scales very easily.
Challenges of ELT
- Can be expensive – ELT tools and resources can be more expensive than ETL tools because more information is processed in less time. ELT can also require more skill than an ETL solution, which may necessitate hiring ELT experts.
ETL vs. ELT: a side-by-side comparison
The following table provides a side-by-side look at how ETL and ELT compare across key factors like cost, complexity, and maintenance time.
Factor/Consideration | ETL | ELT |
Data set size | Small | Large |
Time – transformation | Time intensive, as the transformation process must be fully complete prior to loading | Can take less time since the data can be transformed on an as-needed basis |
Time – loading | Slow | Fast, as the raw data is loaded directly into the target system |
Maintenance time | High maintenance | Low maintenance, but may initially require extra expertise |
Privacy | Pre-loading redactions and transformations keep data private | More privacy safeguards are required |
Data types supported | Structured data | Raw, unstructured, semi-structured, and structured data |
Data lake support | No, ETL isn’t compatible with data lakes | Yes, ELT is compatible with data lakes |
Data warehouse support | Only used for on-premise, relational data | Used in cloud infrastructure |
Cost | Separate servers can create cost issues, but the lower data complexity can offset costs | Can be expensive depending on processing and expertise needs, but the simplified data stack can offset costs |
ETL vs. ELT: which one is right for you?
ETL and ELT are both viable solutions for data movement and transformation but are best suited to different business use cases.
ETL may be best if you:
- Aren’t using real-time data.
- Have smaller datasets that don’t require complex transformations.
- Must reduce any risk of exposing non-compliant data.
- Use an OLAP relational database or SQL data warehousing.
- Do not need to pass unstructured data into a target data system.
- Are willing to wait for long load times.
ELT may be best if you:
- Want to capture all structured and unstructured data, regardless of size, that your business has access to.
- Capture data in real time.
- Have the resources to manage data lakes and hire ELT experts.
- Have a powerful, cloud-based target data system to process incoming data volumes.
- Prioritize fast data loading.
Make data migration easy with SnapLogic ELT
Trustworthy migration and transformation processes fuel trustworthy data. And whether you choose ETL or ELT, the ability to trust and readily access your data is critical to the success of your business. SnapLogic delivers all of these data movement and transformation methods in a proven and powerful cloud-based platform that addresses the unique needs and goals of your organization.
SnapLogic’s iPaaS (integration platform as a service) can help you simplify and automate data and application integration. The iPaaS uses API endpoints that allow different applications within your organization to share information across a common communication channel, enabling more collaborative workflows.
While most iPaaS solutions can integrate with cloud, on-premise, and hybrid systems, SnapLogic also offers a dedicated ELT solution. SnapLogic ELT is a low-code/no-code platform that gives you the flexibility to leverage the power of a cloud data warehouse to transform your data. The tool uses a visual programming language to load data quickly and transform it in place. At the same time, it leverages the cloud data warehouse’s computing power to prepare structured data for analysis.
With more than 700+ pre-built Snaps, SnapLogic powers data ingestion from application and data endpoints. Meanwhile, ELT capabilities within the platform manage transformation through cloud data warehouses like Snowflake, Redshift, Azure Synapse, Google BigQuery, and Databricks Delta Lake. This lets the platform extract summarized data from a cloud data warehouse to any application or analytics endpoint, allowing for greater insights into load efficiency, compute consumption, data volume trends, and more.
Additional FAQs
1. How does the choice between ETL and ELT impact data privacy and compliance?
In the context of data privacy and compliance, organizations employing ETL should focus on implementing robust redaction and encryption measures during the transformation process. It’s crucial to define and adhere to compliance standards such as HIPAA, CCPA, or GDPR, ensuring that sensitive data is handled securely. For ELT, emphasis should be placed on encrypting and protecting data once it’s loaded into the warehouse, considering the potential exposure of all data. Establishing comprehensive data governance policies is essential to mitigate privacy risks in both ETL and ELT processes.
2. What are the key differences in terms of skill requirements for implementing ETL and ELT solutions?
The skills required for ETL predominantly involve expertise in data transformation, database management, and knowledge of ETL tools. On the other hand, ELT demands proficiency in managing data lakes, cloud-based data warehouses, and understanding modern cloud server technologies. Organizations opting for ELT should consider hiring experts familiar with cloud platforms like Amazon Redshift, Snowflake, or Microsoft Azure. While ETL expertise is widespread among developers due to its long-standing presence, ELT may require a more specialized skill set, impacting initial costs but potentially leading to greater efficiency.
3. How should organizations decide between ETL and ELT based on their data characteristics?
When deciding between ETL and ELT based on data characteristics, organizations should assess factors such as data volume, real-time processing needs, and the nature of the target data system. ETL is preferable for organizations with smaller datasets, non-real-time data requirements, and those using on-premise or SQL data warehousing. ELT is suitable for scenarios where capturing all structured and unstructured data, real-time processing, and cloud-based data warehousing are priorities. Understanding specific data use cases, scalability requirements, and processing speed preferences will guide organizations towards the most suitable choice between ETL and ELT.